Learn why more independent practices trust practice fusion than any other cloud-based ehr. we provide intuitive charting with flexible templates and efficient e-prescriptions. Using electronic health records and machine learning to predict postpartum depression postpartum depression (ppd) is one of the most frequent maternal morbidities after delivery with serious implications. currently, there is a lack of effective screening strategies and machine electronic health learning records high-quality clinical trials.
Garg r, dong s, shah s, jonnalagadda sr. a bootstrap machine learning approach to identify rare disease patients from electronic health records. arxiv preprint arxiv:160901586. 2016. 5. colbaugh r, glass k, rudolf c, global mtv. learning to identify rare disease patients from electronic health records. in: amia annual symposium proceedings. Using several machine learning tools, wong et al 1 predicted delirium risk for newly hospitalized patients with high-dimensional electronic health record data at a large academic health institution. they compared these approaches with a questionnaire-based scoring system and found improved performance for machine learning with respect to several metrics calculated in a single holdout sample.
Search machine electronic health learning records for learning machine. find it here! search for learning machine with us. Feb 1, 2020 artificial intelligence in the form of machine learning—which allows computers to identify patterns in data and draw conclusions on their . Aug 3, 2018 machine learning for prediction in electronic health data has been deployed for many clinical questions during the last decade. machine .
Healthcare And Data Science How Ehr And Ai Can Go Handin
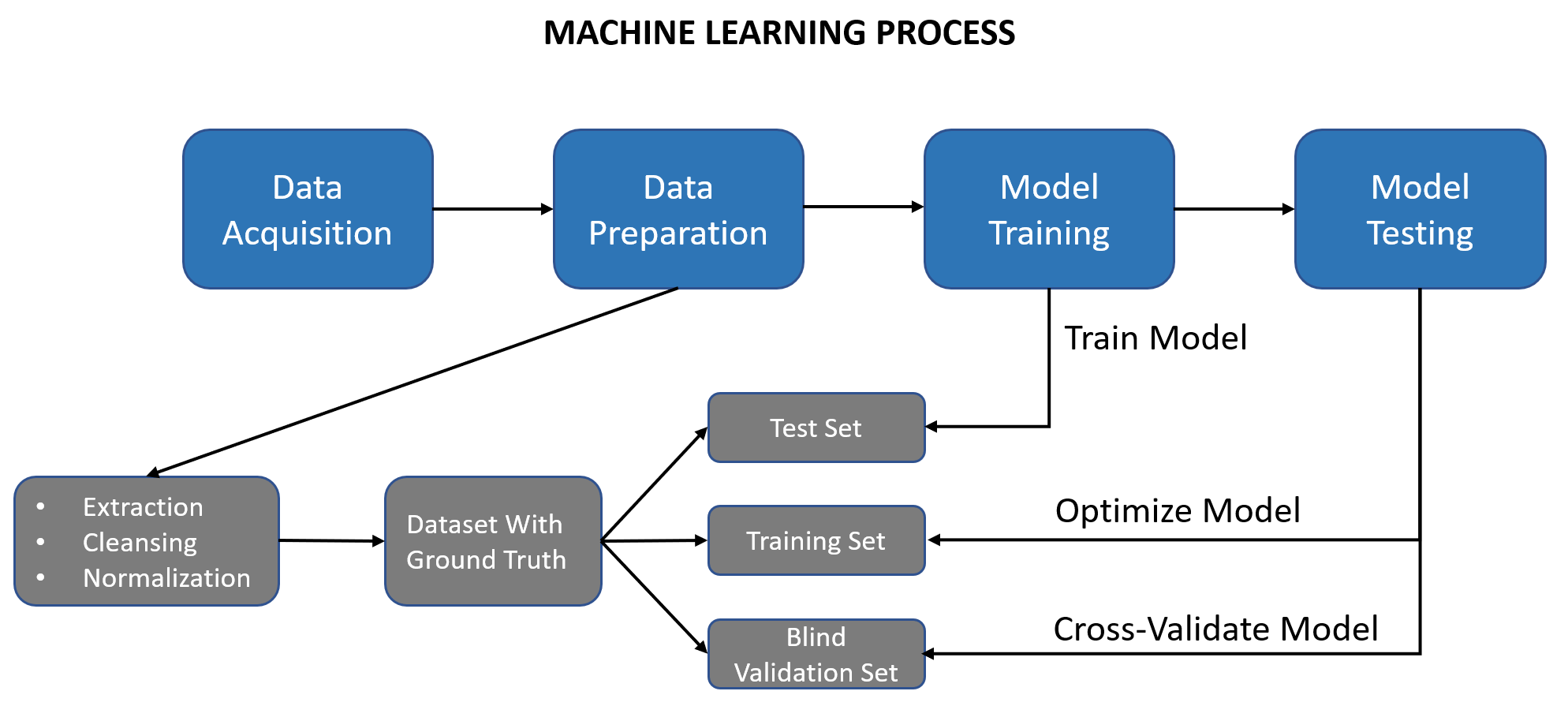
In this way, machine learning algorithms, play a major role in revolutionizing the growing electronic health records. future scope of machine learning in revolutionizing health data and its services with the data analytics is already put to work as the electronic health records were introduced, the future of the healthcare market lies in the fact, that how soon we overcome the challenges of optimizing data and move ahead. the sooner the better. Search for electronic health records. smart results today!. Aug 3, 2020 abstract objective to identify depression subphenotypes from electronic health records (ehrs) using machine learning methods, and . Over the past decade, hospitals and other health care providers have put massive amounts of time and energy into adopting electronic health care records, turning hastily scribbled doctors' notes into durable sources of information. but collecting these data is less than half the battle. it can take even more time and effort to turn these records into actual insights—ones that use the.
Mar 30, 2020 additionally, healthcare provides the perfect input for artificial intelligence (ai) and machine learning (ml) algorithms. streamlined workflows . In this way, machine learning algorithms, play a major role in revolutionizing the growing electronic health records. future scope of machine learning in revolutionizing health data and its services with the data analytics is already put to work as the electronic health records were introduced, the future of the healthcare market lies in the. Machine learning and electronic health records: a paradigm shift. daniel e. adkins, ph. d. in this issue of the journal, barack-corren et al. (1) use. machine .
Following the general trend, the amount of digital information in the stored electronic health records (ehrs) had an explosion in the last decade. ehrs are not. Deep learning (dl) is becoming the main way to study electronic health records (ehr). •. the first comparative review of the key dl architectures used for ehr .
Deep Learning Techniques For Electronic Health Record Analysis
Find electronic health records. get high level results!.
Dec 31, 2019 the terms “electronic medical records or emr or electronic health records or ehr and (artificial intelligence or machine learning or . Electronic health machine electronic health learning records record (ehr) data from millions of patients are now routinely collected across diverse healthcare institutions. they consist of heterogeneous data .
Machine Learning For Prediction In Electronic Health Data
Machine learning on electronic health records: models and.
Shepherding medical data machine learning platform turns healthcare data into insights over the past decade, hospitals and other healthcare providers have put massive amounts of time and energy into adopting electronic healthcare records, turning hastily scribbled doctors' notes into durable sources of information. but collecting these data is. The adoption of electronic health records and the implementation of machine learning elevates healthcare operations to a new level. on the one hand, it expands the view on patient data and puts it into the broader context of healthcare proceedings. The growing adoption of the electronic health record (ehr) worldwide has created new opportunities for leveraging ehr data for other, so called secondary purposes, such as clinical and translational research, quality measurement and improvement, patient cohort identification and more [ 1 ].
Machine learning has generated substantial advances in medical imaging, for example, through improved detection of colonic polyps, cerebral microbleeding, and diabetic retinopathy. 2 predictive modeling with electronic health records using deep learning can accurately predict in-hospital mortality, 30-day unplanned readmission, prolonged length of stay, and final discharge diagnoses. 3. Sources: a medline search was performed with the keywords artificial intelligence, machine learning, infection∗, and infectious disease∗ for the years 2014-2019. studies using routinely available electronic hospital record data from an inpatient setting with a focus on bacterial and fungal infections were included.
Nov 15, 2019 several clinical code representation forms have been proposed by various deep learning ehr systems that share themselves easily to cross . Electronic health record–derived data and novel analytics, such as machine learning, offer promising approaches to identify high-risk patients and inform nursing practice. purpose: the aim was to identify patients at risk for readmissions by applying a machine-learning technique, classification and regression tree, to electronic health record data from our 300-bed hospital. In this issue of the journal, barack-corren et al. use machine learning methods to build a highly predictive model of suicidal behavior using longitudinal electronic health records (ehrs). they do so using a well-established probability-based machine learning algorithm, the naive bayesian classifier, to mine through approximately 1. 7 million patient records, spanning 15 years (1998–2012. Machine learning on electronic health records: models and features usages to predict medication non-adherence. 11/29/2018 ∙ by thomas janssoone, et al. ∙ sêmeia ∙ 0 ∙ share adherence can be defined as "the extent to which patients take their med.